
Bundling Biotech Innovation: How Financial Engineering Can Save Science — and Lives
The time is ripe for new financial solutions to reshape the biotech industry.
One voice at the forefront of financial innovation in biotech is an MIT professor, researcher, and economist named Andrew W. Lo. In recent years, Lo has called attention to a worrying trend in science finance: Even as humans get smarter and technology becomes more powerful, our ability to turn that intelligence into efficient and profitable biomedical outcomes has dwindled.
Part of the problem is our capacity to evaluate risk vs. reward in biotech, which has been steadily declining over the last decade, making it harder to raise money for the early, crucial part of drug discovery.
The science of risk
A research paper by Lo and his MIT colleague, Richard T. Thakor, titled “Financing Biomedical Innovation”, presents unique paths toward advancing biomedicine and early-stage drug development through financial engineering.
In the paper, Lo and Thakor discuss the long, expensive, and risky journey drugs must take to approval, and how unpredictable market conditions have made capital for biopharma firms, especially smaller ones, increasingly scarce. “During market downturns, it will typically be harder for biopharma firms to finance their drug development programs. This realization suggests that the smaller cash-poor biotech companies are much more sensitive to financing risk,” they write.
This friction and the unpredictable nature of funding and clinical trial outcomes has led to “underinvestment in R&D and therapies that are potentially valuable from a societal perspective.”
Lo and Thakor present solutions addressing the high-risk, high-cost challenges of drug development, so that more funding can be “channeled toward drug development, particularly in areas where there may be greater societal need but higher risks for firms.”
One such solution presented by Lo and Thakor is the idea of a so-called “megafund”, which bundles many different biotech projects into a single fund. The idea relies on basic financial portfolio theory and the notion of diversification, such that returns from a small number of successful drugs can offset the individual losses of failed drugs, reducing the overall risk of the fund.
A megafund might focus on a specific scientific field, like cancer research or genetic disorders. Such a fund could enable attractive risk-adjusted returns for both institutions and retail investors who are looking to support cures to specific diseases or solutions that advance specific scientific missions. The key is in the numbers: many projects, one fund, shared risk, and collective reward.

Lo presents an example of a megafund of 150 biomedical research attempts, which, if you multiply the estimated $200 million needed for scientific funding by 150, would require a cool $30 billion to fund—no small potatoes.
But, with that $30 billion spread across 150 individual attempts, the probability of getting at least three successful treatments to completion is 98% (or a .34% Sharpe Ratio). At an estimated $12.3 billion in value per success, that’s a total value of $36.9 billion. Lo’s models, based on historical data, indicate a $5 to $15 billion megafund could yield 9 to 12 percent returns for equity investors.
Not bad – but, where do the billions in scientific funding come from?
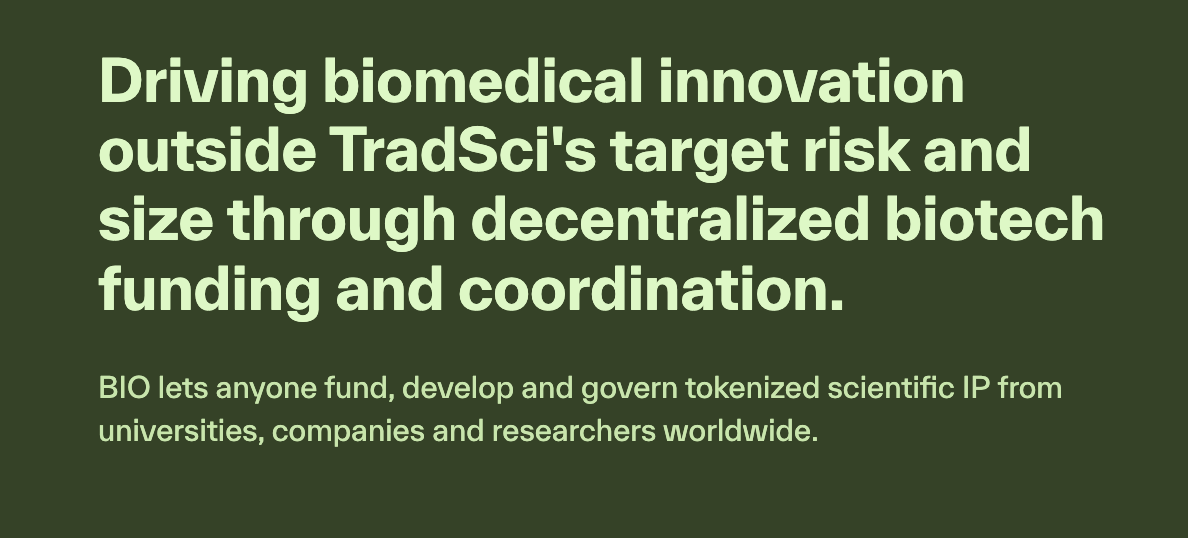
Finding inspiration in the megafund
Lo’s megafund concept speaks to how decentralized funding can be used to de-risk and innovate historically high-risk industries like biomedicine.
The convergence of biotech, AI and crypto technologies can make biotech financing and drug discovery more accessible for institutions as well as global communities of individuals with vested interests in solving specific diseases or global problems, such as patient and advocacy groups.
Our vision for the BIO protocol takes inspiration from these novel models for decentralized funding and financial experimentation. Crypto technologies have the potential to source the needed capital by efficiently pooling funds from a diverse range of globally distributed participants. Transparent financial instruments can then be built atop these pools of capital, allowing for varying levels of publicly scrutable risk. AI can be used to map scientific fields and integrate R&D data models to ensure sufficient diversification across a broad range of promising projects.
In conclusion, we may finally have the financial and open-source tools to realize Lo's long-envisioned megafund concept. Such an infusion of liquidity can accelerate not dozens, but thousands of scientific projects. While many will fail, if even a few drugs are successes, everyone has a better chance to win—and even live. That’s innovation.
Further Reading:
- Financial Biomedical Innovation, Lo & Thakor
- Watch: Can Financial Engineering Cure Cancer?
- Commercializing Biomedical Research through Securitization Techniques (Nature)
- Megafund Shows Why Conventional Thinking Won’t Fix Pharma’s Innovation Crisis
- Introducing BIO: Your Gateway to Decentralized Science